[Update 2017.06.11] Add chainer v2 code
Writing your CNN model
This is example of small Convolutional Neural Network definition, CNNSmall
import chainer import chainer.functions as F import chainer.links as L class CNNSmall(chainer.Chain): def __init__(self, n_out): super(CNNSmall, self).__init__() with self.init_scope(): self.conv1 = L.Convolution2D(None, 16, 3, 2) self.conv2 = L.Convolution2D(16, 32, 3, 2) self.conv3 = L.Convolution2D(32, 32, 3, 2) self.fc4 = L.Linear(None, 100) self.fc5 = L.Linear(100, n_out) def __call__(self, x): h = F.relu(self.conv1(x)) h = F.relu(self.conv2(h)) h = F.relu(self.conv3(h)) h = F.relu(self.fc4(h)) h = self.fc5(h) return h
I also made a slightly bigger CNN, called CNNMedium,
import chainer import chainer.functions as F import chainer.links as L class CNNMedium(chainer.Chain): def __init__(self, n_out): super(CNNMedium, self).__init__() with self.init_scope(): self.conv1 = L.Convolution2D(None, 16, 3, 1) self.conv2 = L.Convolution2D(16, 32, 3, 2) self.conv3 = L.Convolution2D(32, 32, 3, 1) self.conv4 = L.Convolution2D(32, 64, 3, 2) self.conv5 = L.Convolution2D(64, 64, 3, 1) self.conv6 = L.Convolution2D(64, 128, 3, 2) self.fc7 = L.Linear(None, 100) self.fc8 = L.Linear(100, n_out) def __call__(self, x): h = F.relu(self.conv1(x)) h = F.relu(self.conv2(h)) h = F.relu(self.conv3(h)) h = F.relu(self.conv4(h)) h = F.relu(self.conv5(h)) h = F.relu(self.conv6(h)) h = F.relu(self.fc7(h)) h = self.fc8(h) return h
It is nice to know the computational cost for Convolution layer, which is approximated as,
- \( CH_I \) : Input image channel
- \( CH_O \) : Output image channel
- \( H_I \) : Input image height
- \( W_I \) : Input image width
- \( k \) : kernal size (assuming same for width & height)
In above CNN definitions, the size of the channel is bigger for deeper layer. This can be understood by calculating the computational cost for each layer.
When L.Convolution2D
with stride=2 is used, the size of image become almost half. This means \( H_I\) and \( W_I \) becomes small value, so \(CH_I \) and \( CH_O \) can take larger value.
[TODO: add computational cost table for CNN Medium example]
Training CIFAR-10
Once you have written CNN, it is easy to train this model. The code, train_cifar10.py, is quite similar to MNIST training code.
Only small difference is the dataset preparation for CIFAR-10,
# 3. Load the CIFAR-10 dataset train, test = chainer.datasets.get_cifar10()
and model setup
from CNNSmall import CNNSmall from CNNMedium import CNNMedium archs = { 'cnnsmall': CNNSmall, 'cnnmedium': CNNMedium, } ... class_num = 10 model = archs[args.arch](n_out=class_num)
The whole source code is the following,
from __future__ import print_function import argparse import chainer import chainer.functions as F import chainer.links as L from chainer import training, iterators, serializers, optimizers from chainer.training import extensions from CNNSmall import CNNSmall from CNNMedium import CNNMedium def main(): archs = { 'cnnsmall': CNNSmall, 'cnnmedium': CNNMedium, } parser = argparse.ArgumentParser(description='Cifar-10 CNN example') parser.add_argument('--arch', '-a', choices=archs.keys(), default='cnnsmall', help='Convnet architecture') parser.add_argument('--batchsize', '-b', type=int, default=64, help='Number of images in each mini-batch') parser.add_argument('--epoch', '-e', type=int, default=20, help='Number of sweeps over the dataset to train') parser.add_argument('--gpu', '-g', type=int, default=-1, help='GPU ID (negative value indicates CPU)') parser.add_argument('--out', '-o', default='result-cifar10', help='Directory to output the result') parser.add_argument('--resume', '-r', default='', help='Resume the training from snapshot') args = parser.parse_args() print('GPU: {}'.format(args.gpu)) print('# Minibatch-size: {}'.format(args.batchsize)) print('# epoch: {}'.format(args.epoch)) print('') # 1. Setup model class_num = 10 model = archs[args.arch](n_out=class_num) classifier_model = L.Classifier(model) if args.gpu >= 0: chainer.cuda.get_device(args.gpu).use() # Make a specified GPU current classifier_model.to_gpu() # Copy the model to the GPU # 2. Setup an optimizer optimizer = optimizers.Adam() optimizer.setup(classifier_model) # 3. Load the CIFAR-10 dataset train, test = chainer.datasets.get_cifar10() # 4. Setup an Iterator train_iter = iterators.SerialIterator(train, args.batchsize) test_iter = iterators.SerialIterator(test, args.batchsize, repeat=False, shuffle=False) # 5. Setup an Updater updater = training.StandardUpdater(train_iter, optimizer, device=args.gpu) # 6. Setup a trainer (and extensions) trainer = training.Trainer(updater, (args.epoch, 'epoch'), out=args.out) # Evaluate the model with the test dataset for each epoch trainer.extend(extensions.Evaluator(test_iter, classifier_model, device=args.gpu)) trainer.extend(extensions.dump_graph('main/loss')) trainer.extend(extensions.snapshot(), trigger=(1, 'epoch')) trainer.extend(extensions.LogReport()) trainer.extend(extensions.PrintReport( ['epoch', 'main/loss', 'validation/main/loss', 'main/accuracy', 'validation/main/accuracy', 'elapsed_time'])) trainer.extend(extensions.PlotReport( ['main/loss', 'validation/main/loss'], x_key='epoch', file_name='loss.png')) trainer.extend(extensions.PlotReport( ['main/accuracy', 'validation/main/accuracy'], x_key='epoch', file_name='accuracy.png')) trainer.extend(extensions.ProgressBar()) # Resume from a snapshot if args.resume: serializers.load_npz(args.resume, trainer) # Run the training trainer.run() serializers.save_npz('{}/{}-cifar10.model' .format(args.out, args.arch), model) if __name__ == '__main__': main()
See how clean the code is! Chainer abstracts the training process and thus the code can be reusable with other deep learning training.
[hands on] Try running train code.
Below is example in my environment
- CNNSmall model
$ python train_cifar10.py -g 0 -o result-cifar10-cnnsmall -a cnnsmall GPU: 0 # Minibatch-size: 64 # epoch: 20 epoch main/loss validation/main/loss main/accuracy validation/main/accuracy elapsed_time 1 1.66603 1.44016 0.397638 0.477807 6.22123 2 1.36101 1.31731 0.511324 0.527568 12.0878 3 1.23553 1.20439 0.559119 0.568073 17.9239 4 1.14553 1.13121 0.589609 0.595541 23.7497 5 1.08058 1.09946 0.617747 0.606588 29.5948 6 1.02242 1.1259 0.638784 0.605295 35.4604 7 0.97847 1.0797 0.65533 0.615048 41.3058 8 0.938967 1.0584 0.669494 0.621815 47.184 9 0.902363 1.00883 0.681985 0.646099 53.0965 10 0.872796 1.00743 0.692782 0.644904 58.982 11 0.838787 0.993791 0.705226 0.651971 64.9511 12 0.813549 0.987916 0.714609 0.655454 70.3869 13 0.785552 0.987968 0.723825 0.659236 75.8247 14 0.766127 1.0092 0.730074 0.656748 81.4311 15 0.743967 1.04623 0.738496 0.650876 86.9175 16 0.723779 0.991238 0.744518 0.665008 92.6226 17 0.704939 1.02468 0.752058 0.655354 98.1399 18 0.68687 0.999966 0.756962 0.660629 103.657 19 0.668204 1.00803 0.763564 0.660928 109.226 20 0.650081 1.01554 0.769906 0.667197 114.705
Chainer extension, PlotReport
will automatically create the graph of loss and accuracy for each epoch.
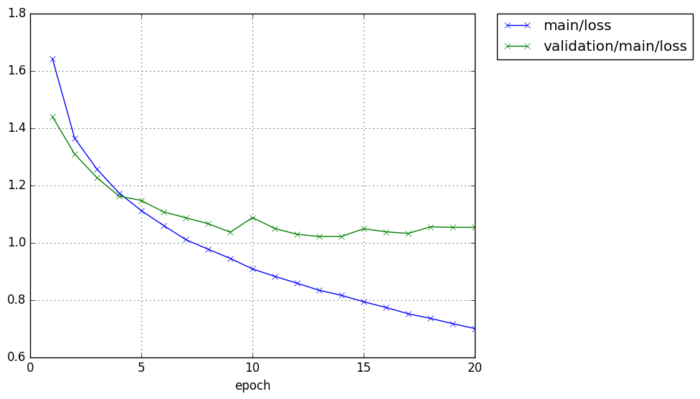
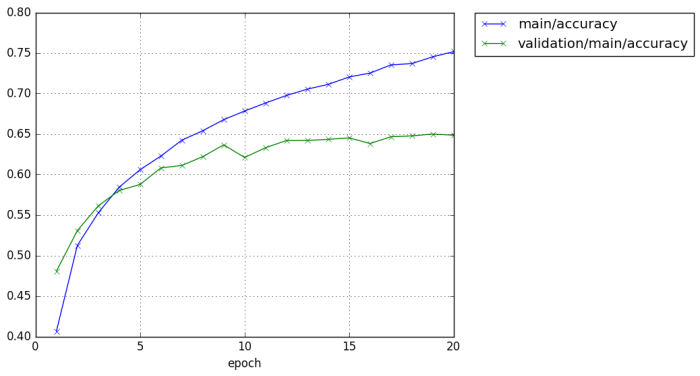
We can achieve around 65% validation accuracy with such a easy CNN construction.
- CNNMedium
$ python train_cifar10.py -g 0 -o result-cifar10-cnnmedium -a cnnmedium GPU: 0 # Minibatch-size: 64 # epoch: 20 epoch main/loss validation/main/loss main/accuracy validation/main/accuracy elapsed_time 1 1.62656 1.3921 0.402494 0.493133 7.61706 2 1.31508 1.2771 0.526448 0.54588 15.209 3 1.14961 1.12021 0.589749 0.603603 22.7185 4 1.04442 1.05119 0.631182 0.629877 30.1564 5 0.947944 1.00655 0.66624 0.648288 37.8547 6 0.876341 1.0247 0.690021 0.644705 46.9253 7 0.819997 0.983303 0.711968 0.662719 54.9994 8 0.757557 0.933339 0.733795 0.677846 62.4761 9 0.699673 0.948701 0.751539 0.682126 69.8784 10 0.652811 0.965453 0.769006 0.680533 77.2829 11 0.606698 0.990516 0.785551 0.671278 84.6915 12 0.559568 0.999138 0.799996 0.682822 92.068 13 0.521884 1.07451 0.814158 0.678742 99.4703 14 0.477247 1.08184 0.829445 0.673865 107.249 15 0.443625 1.08582 0.840109 0.680832 114.609 16 0.406318 1.26192 0.853573 0.660529 122.218 17 0.378328 1.2075 0.86507 0.670183 129.655 18 0.349719 1.27795 0.87548 0.673467 137.098 19 0.329299 1.32094 0.881702 0.664709 144.553 20 0.297305 1.39914 0.894426 0.666202 151.959
As expected, CNNMedium takes little bit longer time for computation but it achieves higher accuracy for training data.
※ It is also important to notice that validation accuracy is almost same between CNNSmall and CNNMedium, which means CNNMedium may be overfitting to the training data. To avoid overfitting, data augmentation (flip, rotate, clip, resize, add gaussian noise etc the input image to increase the effective data size) technique is often used in practice.
Training CIFAR-100
Again, training CIFAR-100 is quite similar to the training of CIFAR-10.
See train_cifar100.py. Only the difference is model definition to set the output class number (model definition itself is not changed and can be reused!!).
# 1. Setup model class_num = 100 model = archs[args.arch](n_out=class_num)
and dataset preparation
# 3. Load the CIFAR-10 dataset train, test = chainer.datasets.get_cifar100()
[hands on] Try running train code.
Summary
We have learned how to train CNN with Chainer. CNN is widely used many image processing tasks, not only image classification. For example,
- Bounding Box detection
- SSD, YoLo V2
- Semantic segmentation
- FCN
- Colorization
- PaintsChainer
- Image generation
- GAN
- Style transfer
- chainer goph
- Super resolution
- SeRanet
etc. Now you are ready to enter these advanced image processing with deep learning!
[hands on]
Try modifying the CNN model or create your own CNN model and train it to see the computational speed and its performance (accuracy). You may try changing following
- model depth
- channel size of each layer
- Layer (Ex. use
F.max_pooling_2d
instead ofL.Convolution2D
with stride 2) - activation function (
F.relu
toF.leaky_relu
,F.sigmoid
,F.tanh
etc…) - Try inserting another layer, Ex.
L.BatchNormalization
orF.dropout
.
etc.
You can refer Chainer example codes to see the network definition examples.
Also, try configuring hyper parameter to see the performance
- Change optimizer
- Change learning rate of optimizer
etc.