Basic image processing for deep learning. Refer github for the source code.
The sample image is obtained from PEXELS.
If you are not familiar with image processing, you can read this article before going to convolutional neural network.
OpenCV is image processing library which supports
- loading image in numpy.ndarray format, save image
- converting image color format (RGB, YUV, Gray scale etc)
- resize
and other useful image processing functionality.
To install opencv, execute
$conda install -c https://conda.binstar.org/menpo -y opencv3
import os import matplotlib.pyplot as plt import cv2 %matplotlib inline def readRGBImage(imagepath): image = cv2.imread(imagepath) # Height, Width, Channel (major, minor, _) = cv2.__version__.split(".") if major == '3': # version 3 is used, need to convert image = cv2.cvtColor(image, cv2.COLOR_BGR2RGB) else: # Version 2 is used, not necessary to convert pass return image
Loading and save image
cv2.imread
for loading image.cv2.imwrite
for save image.plt.imshow
for plotting, andplt.savefig
for save plot image.
OpenCV image format is usually 3 dimension (or 2 dimension if the image is gray scale).
1st dimension is for height, 2nd dimension is for width, 3rd dimension is for channel (RGB, YUV etc).
To convert color format cv2.cvtColor
can be used. Details are written in next section.
# Read image from file, save image with matplotlib using `imshow` function basedir = './src/cnn/images' imagepath = os.path.join(basedir, 'sample.jpeg') #image = cv2.imread(imagepath, cv2.IMREAD_GRAYSCALE) image = readRGBImage(imagepath) # Width and Height shows pixel size of this image # Channel=3 indicates the RGB channel print('image.shape (Height, Width, Channel) = ', image.shape) # Save image with openCV # This may be blue image because the color format RGB is opposite. cv2.imwrite('./src/cnn/images/out.jpg', image) # bgr_image = cv2.cvtColor(image, cv2.COLOR_RGB2BGR) # cv2.imwrite('./src/cnn/images/out.jpg', bgr_image) # Plotting plt.imshow(image) plt.savefig('./src/cnn/images/out_plt.png')
image.shape (Height, Width, Channel) = (380, 512, 3)
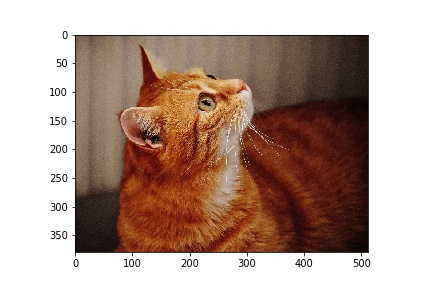
Change color format
cv2.cvtColor
for converting color format.
Note that openCV version 3 reads the image color in the order B, G, R. However, matplotlib deals with the image color in the corder R, G, B. So you need to convert color order, refer readRGBImage
function.
If the image is gray scale, the image is 2 dimensional array
1st dimension is for height, 2nd dimension is for width.
gray_image = cv2.cvtColor(image, cv2.COLOR_RGB2GRAY) # Gray scale image is 2 dimension, No channel dimension. print('gray_image.shape (Height, Width) = ', gray_image.shape) cv2.imwrite('./src/cnn/images/out_gray.jpg', gray_image)
gray_image.shape (Height, Width) = (380, 512)
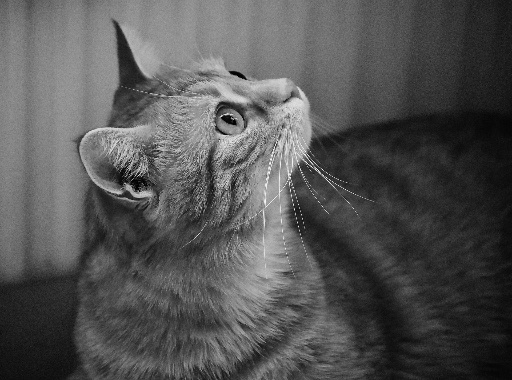
Resize
cv2.imread
for resize.
Note that size should be specified in the order width, height.
%matplotlib inline print('image.shape (Height, Width, Channel) = ', image.shape) # Resize image to half size height, width = image.shape[:2] half_image = cv2.resize(image, (width//2, height//2)) # size must be int print('half_image.shape (Height, Width, Channel) = ', half_image.shape) plt.imshow(half_image) plt.savefig('./src/cnn/images/out_half.jpeg') # Resize image by specifying longer side size def resize_longedge(image, pixel): """Resize the input image Longer edge size will be `pixel`, and aspect ratio doesn't change """ height, width = image.shape[:2] longer_side = max(height, width) ratio = float(pixel) / longer_side return cv2.resize(image, None, fx=ratio, fy=ratio) # size must be int resized128_image = resize_longedge(image, 128) print('resized128_image.shape (Height, Width, Channel) = ', resized128_image.shape) plt.imshow(resized128_image) plt.savefig('./src/cnn/images/out_resized128.jpg')
image.shape (Height, Width, Channel) = (380, 512, 3)
half_image.shape (Height, Width, Channel) = (190, 256, 3)
resized128_image.shape (Height, Width, Channel) = (95, 128, 3)
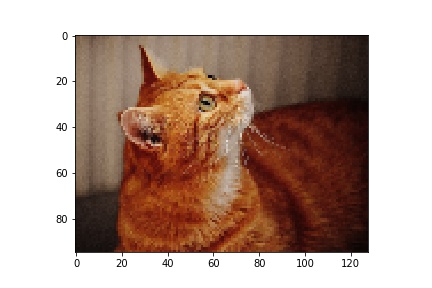
Crop
- numpy slicing can be used for cropping image
# Crop center of half_image height, width = half_image.shape[:2] crop_length = min(height, width) height_start = (height - crop_length) // 2 width_start = (width - crop_length) // 2 cropped_image = half_image[ height_start:height_start+crop_length, width_start:width_start+crop_length, :] print('cropped_image.shape (Height, Width, Channel) = ', cropped_image.shape) plt.imshow(cropped_image) plt.savefig('./src/cnn/images/out_cropped.jpg')
cropped_image.shape (Height, Width, Channel) = (190, 190, 3)
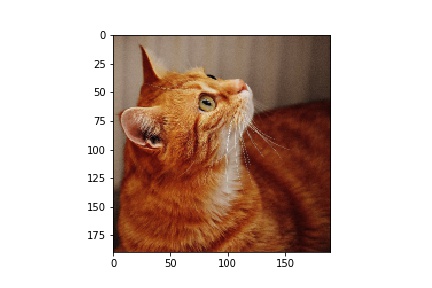
Image processing with channels
RGB channel manipulation.
Understanding the meaning of “channel” is important in deep learning. Below code provides some insight that what each channel represents.
%matplotlib inline # Show RGB channel separately in gray scale fig, axes = plt.subplots(1, 3) # image[:, :, 0] is R channel. axes[0].set_title('R channel') axes[0].imshow(image[:, :, 0], cmap='gray') # image[:, :, 1] is G channel. axes[1].set_title('G channel') axes[1].imshow(image[:, :, 1], cmap='gray') # image[:, :, 2] is B channel. axes[2].set_title('B channel') axes[2].imshow(image[:, :, 2], cmap='gray') plt.savefig(os.path.join(basedir, 'RGB_gray.jpg'))
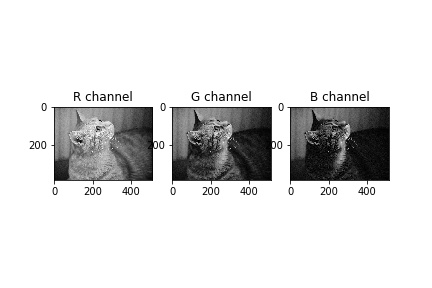
# Show RGB channel separately in color fig, axes = plt.subplots(1, 3) # image[:, :, 0] is R channel, replace the rest by 0. imageR = image.copy() imageR[:, :, 1:3] = 0 axes[0].set_title('R channel') axes[0].imshow(imageR) # image[:, :, 1] is G channel, replace the rest by 0. imageG = image.copy() imageG[:, :, [0, 2]] = 0 axes[1].set_title('G channel') axes[1].imshow(imageG) # image[:, :, 2] is B channel, replace the rest by 0. imageB = image.copy() imageB[:, :, 0:2] = 0 axes[2].set_title('B channel') axes[2].imshow(imageB) plt.savefig(os.path.join(basedir, 'RGB_color.jpg'))
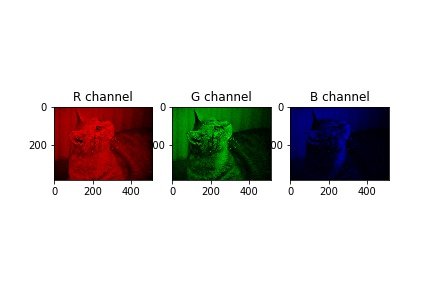