We have learned in previous post that RNN is expected to have an ability to remember the sequence information. Let’s do a easy experiment to check it before trying actual NLP application.
Contents
Simple sequence dataset
I just prepared a simple script to generate simple integer sequence as follows,
- Source code: simple_sequence_dataset.py
import numpy as np N_VOCABULARY = 10 def get_simple_sequence(n_vocab, repeat=100): data = [] for i in range(repeat): for j in range(n_vocab): for k in range(j): data.append(j) return np.asarray(data, dtype=np.int32) if __name__ == '__main__': data = get_simple_sequence(N_VOCABULARY) print(data)
Its output is,
[1 2 2 3 3 3 4 4 4 4 5 5 5 5 5 6 6 6 6 6 6 7 7 7 7 7 7 7 8 8 8 8 8 8 8 8 9 9 9 9 9 9 9 9 9 1 2 2 ..., 9 9 9]
So the number i is repeated i times. In order for RNN to generate correct sequence, RNN need to “count” how many times this number already appeared.
For example, to output correct sequence of 9 9 9 … followed by 1, RNN need to count if 9 is already appeared 9 times to output 1.
Training code for RNN
Training procedure of RNN is little bit complicated compared to MLP or CNN, due to the existence of recurrent loop and we need to deal with back propagation with sequential data properly.
To achieve this, we implement custom iterator and updater.
※ Following implementation just referenced from Chainer official example code.
Iterator – feed data sequentially
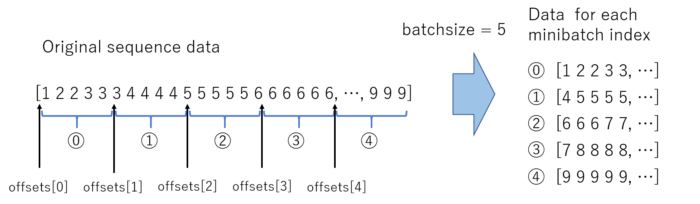
When training RNN, we need to input the data sequentially. Thus we should not take random permutation. We need to be careful when creating the minibatch dataset so that each minibatch should be feed in sequence.
You can implement custom Iterator
class to achieve this functionality. The parent class Iterator
is implemented as following, Iterator code.
So what we need to implement in Iterator
is
__init__(self, ...)
:
Initialization code.__next__(self)
:
This is the core part of iterator. For each iteration, this function is automatically called to get next minibatch.epoch_detail(self)
:
This property is used by trainer module to show the progress of training.serialize(self)
:
Implement if you want to support resume functionality of trainer.
We will implement ParallelSequentialIterator
, works following, please also see the figure above.
- It will get
dataset
in the__init__
code, and split the dataset equally with sizebatch_size
. - Every iteration of the training loop,
__next__()
is called.
This iterator will prepare current word (input data) and next word (answer data).
The RNN model is trained to predict next word from current word (and its recurrent unit, which encodes the past sequence information). - Additionally, in order for the trainer extensions to work nicely,
epoch_detail
andserialize
are implemented. (These are not mandatory for minimum implementation.)
The final code looks like following,
- Source code: parallel_sequential_iterator.py
""" This code is copied from official chainer examples - https://github.com/chainer/chainer/blob/e2fe6f8023e635f8c1fc9c89e85d075ebd50c529/examples/ptb/train_ptb.py """ import chainer # Dataset iterator to create a batch of sequences at different positions. # This iterator returns a pair of current words and the next words. Each # example is a part of sequences starting from the different offsets # equally spaced within the whole sequence. class ParallelSequentialIterator(chainer.dataset.Iterator): def __init__(self, dataset, batch_size, repeat=True): self.dataset = dataset self.batch_size = batch_size # batch size # Number of completed sweeps over the dataset. In this case, it is # incremented if every word is visited at least once after the last # increment. self.epoch = 0 # True if the epoch is incremented at the last iteration. self.is_new_epoch = False self.repeat = repeat length = len(dataset) # Offsets maintain the position of each sequence in the mini-batch. self.offsets = [i * length // batch_size for i in range(batch_size)] # NOTE: this is not a count of parameter updates. It is just a count of # calls of ``__next__``. self.iteration = 0 def __next__(self): # This iterator returns a list representing a mini-batch. Each item # indicates a different position in the original sequence. Each item is # represented by a pair of two word IDs. The first word is at the # "current" position, while the second word at the next position. # At each iteration, the iteration count is incremented, which pushes # forward the "current" position. length = len(self.dataset) if not self.repeat and self.iteration * self.batch_size >= length: # If not self.repeat, this iterator stops at the end of the first # epoch (i.e., when all words are visited once). raise StopIteration cur_words = self.get_words() self.iteration += 1 next_words = self.get_words() epoch = self.iteration * self.batch_size // length self.is_new_epoch = self.epoch < epoch if self.is_new_epoch: self.epoch = epoch return list(zip(cur_words, next_words)) @property def epoch_detail(self): # Floating point version of epoch. return self.iteration * self.batch_size / len(self.dataset) def get_words(self): # It returns a list of current words. return [self.dataset[(offset + self.iteration) % len(self.dataset)] for offset in self.offsets] def serialize(self, serializer): # It is important to serialize the state to be recovered on resume. self.iteration = serializer('iteration', self.iteration) self.epoch = serializer('epoch', self.epoch)
Updater – Truncated back propagation through time (BPTT)
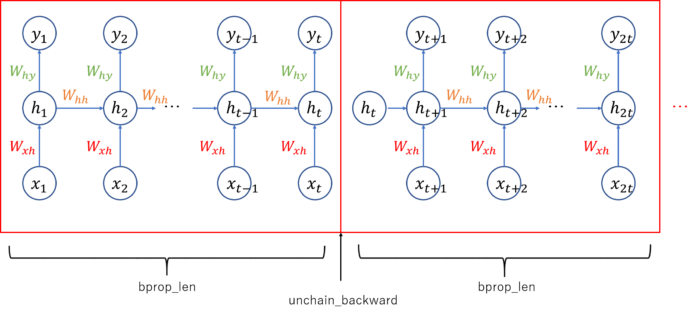
Back propagation through time: The training procedure for RNN model is different from MLP or CNN. Because each forward computation of RNN depends on the previous forward computation due to the existence of recurrent unit. Therefore we need to execute forward
computation several times before executing backward
computation to allow recurrent loop, Whh, to learn the sequential information. We set the value bprop_len
(back propagation length) in below Updater
implementation. Forward computation is executed this number of times consecutively, followed by one time of back propagation.
Truncate computational graph: Also, as you can see from the above figure, RNN graph will grow every time the forward computation is executed, and computer cannot handle if the graph grows infinitely long. To deal with this issue, we will cut (truncate) the graph after each time of backward
computation. It can be achieved by calling unchain_backward
function in chainer.
This optimization method can be implemented by creating custom Updater
class, BPTTUpdater
, as a subclass of StandardUpdater
.
It just overrides the function update_core
, which is the function to write parameter update (optimize) process.
Source code: bptt_updater.py
“””
This code is copied from official chainer examples
- https://github.com/chainer/chainer/blob/e2fe6f8023e635f8c1fc9c89e85d075ebd50c529/examples/ptb/train_ptb.py
“””
import chainer
from chainer import training
Custom updater for truncated BackProp Through Time (BPTT)
class BPTTUpdater(training.StandardUpdater):
def __init__(self, train_iter, optimizer, bprop_len, device): super(BPTTUpdater, self).__init__( train_iter, optimizer, device=device) self.bprop_len = bprop_len # The core part of the update routine can be customized by overriding. def update_core(self): loss = 0 # When we pass one iterator and optimizer to StandardUpdater.__init__, # they are automatically named 'main'. train_iter = self.get_iterator('main') optimizer = self.get_optimizer('main') # Progress the dataset iterator for bprop_len words at each iteration. for i in range(self.bprop_len): # Get the next batch (a list of tuples of two word IDs) batch = train_iter.__next__() # Concatenate the word IDs to matrices and send them to the device # self.converter does this job # (it is chainer.dataset.concat_examples by default) x, t = self.converter(batch, self.device) # Compute the loss at this time step and accumulate it loss += optimizer.target(chainer.Variable(x), chainer.Variable(t)) optimizer.target.cleargrads() # Clear the parameter gradients loss.backward() # Backprop loss.unchain_backward() # Truncate the graph optimizer.update() # Update the parameters
As you can see, forward
is executed in the for loop bprop_len
times consecutively to accumulate loss
, followed by one backward
to execute the back propagation of this accumulated loss
. After that, the parameter is updated by optimizer
using update
funciton.
Note that unchain_backward
is called every time at the end of the update_core
function to truncate/cut the computational graph.
Main training code
Once iterator and the updater are prepared, training code is almost same with previous training for MLP-MNIST task or CNN-CIFAR10/CIFAR100.
- Source code: train_simple_sequence.py
""" RNN Training code with simple sequence dataset """ from __future__ import print_function import os import sys import argparse import matplotlib matplotlib.use('Agg') import matplotlib.pyplot as plt import chainer import chainer.functions as F import chainer.links as L from chainer import training, iterators, serializers, optimizers from chainer.training import extensions sys.path.append(os.pardir) from RNN import RNN from RNN2 import RNN2 from RNN3 import RNN3 from RNNForLM import RNNForLM from simple_sequence.simple_sequence_dataset import N_VOCABULARY, get_simple_sequence from parallel_sequential_iterator import ParallelSequentialIterator from bptt_updater import BPTTUpdater def main(): archs = { 'rnn': RNN, 'rnn2': RNN2, 'rnn3': RNN3, 'lstm': RNNForLM } parser = argparse.ArgumentParser(description='RNN example') parser.add_argument('--arch', '-a', choices=archs.keys(), default='rnn', help='Net architecture') parser.add_argument('--unit', '-u', type=int, default=100, help='Number of RNN units in each layer') parser.add_argument('--bproplen', '-l', type=int, default=20, help='Number of words in each mini-batch ' '(= length of truncated BPTT)') parser.add_argument('--batchsize', '-b', type=int, default=10, help='Number of images in each mini-batch') parser.add_argument('--epoch', '-e', type=int, default=10, help='Number of sweeps over the dataset to train') parser.add_argument('--gpu', '-g', type=int, default=-1, help='GPU ID (negative value indicates CPU)') parser.add_argument('--out', '-o', default='result', help='Directory to output the result') parser.add_argument('--resume', '-r', default='', help='Resume the training from snapshot') args = parser.parse_args() print('GPU: {}'.format(args.gpu)) print('# Architecture: {}'.format(args.arch)) print('# Minibatch-size: {}'.format(args.batchsize)) print('# epoch: {}'.format(args.epoch)) print('') # 1. Setup model #model = archs[args.arch](n_vocab=N_VOCABRARY, n_units=args.unit) # activation=F.leaky_relu model = archs[args.arch](n_vocab=N_VOCABULARY, n_units=args.unit) # , activation=F.tanh classifier_model = L.Classifier(model) if args.gpu >= 0: chainer.cuda.get_device(args.gpu).use() # Make a specified GPU current classifier_model.to_gpu() # Copy the model to the GPU eval_classifier_model = classifier_model.copy() # Model with shared params and distinct states eval_model = classifier_model.predictor # 2. Setup an optimizer optimizer = optimizers.Adam(alpha=0.0005) #optimizer = optimizers.MomentumSGD() optimizer.setup(classifier_model) # 3. Load dataset train = get_simple_sequence(N_VOCABULARY) test = get_simple_sequence(N_VOCABULARY) # 4. Setup an Iterator train_iter = ParallelSequentialIterator(train, args.batchsize) test_iter = ParallelSequentialIterator(test, args.batchsize, repeat=False) # 5. Setup an Updater updater = BPTTUpdater(train_iter, optimizer, args.bproplen, args.gpu) # 6. Setup a trainer (and extensions) trainer = training.Trainer(updater, (args.epoch, 'epoch'), out=args.out) # Evaluate the model with the test dataset for each epoch trainer.extend(extensions.Evaluator(test_iter, eval_classifier_model, device=args.gpu, # Reset the RNN state at the beginning of each evaluation eval_hook=lambda _: eval_model.reset_state()) ) trainer.extend(extensions.dump_graph('main/loss')) trainer.extend(extensions.snapshot(), trigger=(1, 'epoch')) trainer.extend(extensions.LogReport()) trainer.extend(extensions.PrintReport( ['epoch', 'main/loss', 'validation/main/loss', 'main/accuracy', 'validation/main/accuracy', 'elapsed_time'])) trainer.extend(extensions.PlotReport( ['main/loss', 'validation/main/loss'], x_key='epoch', file_name='loss.png')) trainer.extend(extensions.PlotReport( ['main/accuracy', 'validation/main/accuracy'], x_key='epoch', file_name='accuracy.png')) # trainer.extend(extensions.ProgressBar()) # Resume from a snapshot if args.resume: serializers.load_npz(args.resume, trainer) # Run the training trainer.run() serializers.save_npz('{}/{}_simple_sequence.model' .format(args.out, args.arch), model) if __name__ == '__main__': main()
Run the code
You can execute the code like,
python train_simple_sequence.py
You can also train with different model using -a
option,
python train_simple_sequence.py -a rnn2
Below is the result in my environment with RNN
architecture,
GPU: -1 # Architecture: rnn # Minibatch-size: 10 # epoch: 10 epoch main/loss validation/main/loss main/accuracy validation/main/accuracy elapsed_time 1 2.15793 1.04886 0.434783 0.862222 1.11497 2 1.09747 0.569532 0.681818 0.866667 2.63032 3 0.77518 0.4109 0.652174 0.866667 4.14638 4 0.621658 0.335307 0.727273 0.888889 5.66036 5 0.497747 0.278632 0.782609 0.911111 7.15996 6 0.429227 0.233576 0.818182 0.955556 8.61034 7 0.360052 0.194116 0.913043 0.955556 10.0369 8 0.312902 0.162933 0.863636 0.977778 11.4006 9 0.317397 0.141921 0.913043 0.977778 12.8574 10 0.281399 0.120881 0.909091 1 14.215
I set the N_VOCABULARY=10
in simple_sequence_dataset.py
, and even the simple RNN
achieved the accuracy close to 1. It seems this RNN
model have an ability to remember past 10 sequence.